Measuring human perceptions of expressivity in natural and artificial systems through the live…
A research Article Open Access Catie Cuan*, Erin Berl, and Amy LaViers originally published by De Gruyter.
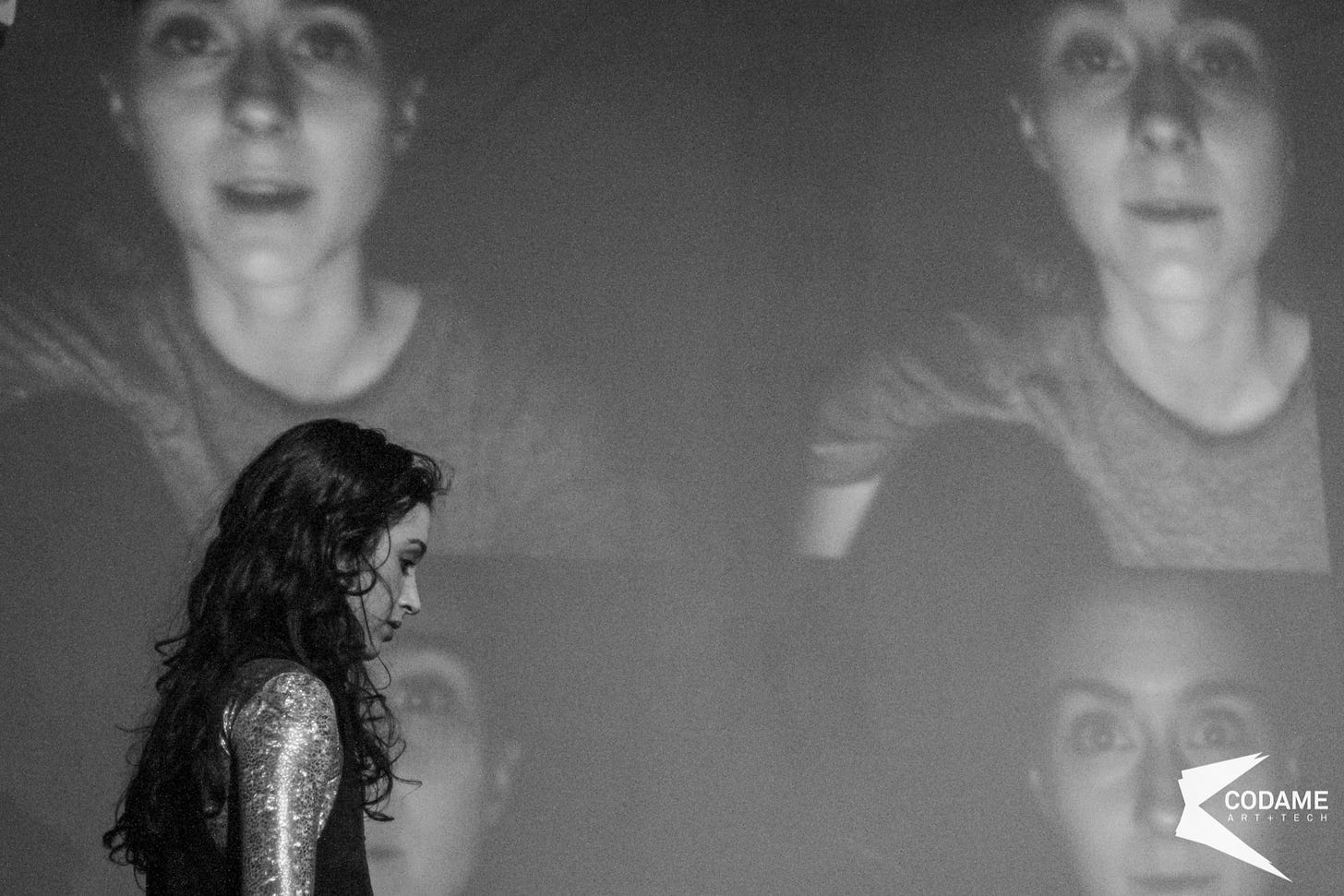
Time to compile at the CODAME ART+TECH Festival (2018) #ArtoBots
Abstract
Live performance is a vehicle where theatrical devices are used to exemplify, probe, or question how humans think about objects, each other, and themselves. This paper presents work using this vehicle to explore human perceptions of robot and human capabilities. The paper documents four performances at three distinct venues where user studies were conducted in parallel to live performance. A set of best practices for successful collection of data in this manner over the course of these trials is developed. Then, results of the studies are presented, giving insight into human opinions of a variety of natural and artificial systems. In particular, participants are asked to rate the expressivity of 12 distinct systems, displayed on stage, as well as themselves. The results show trends ranking objects lowest, then robots, then humans, then self, highest. Moreover, objects involved in the show were generally rated higher after the performance. Qualitative responses give further insight into how viewers experienced watching human performers alongside elements of technology. This work lays a framework for measuring human perceptions of robotic systems and factors that influence this perception inside live performance and suggests black that through the lens of expressivity systems of similar type are rated similarly by audience members.
Introduction
Roboticists have a task to complete in the process of incorporating robots into new, human-facing environments. As artificial, robotic bodies begin to co-exist alongside humans in their daily lives, a new story is written. What will people think about these new counterparts? How will we feel? What new ideas will these distinct bodies spark for humans and how will we make sense of them? How will we judge ourselves in new environments alongside machine counterparts?
Historically, the answer to these questions have been answered by artists. When Martha Graham used deep, bended contractions to express the struggle of the Modern Era, she helped people value and exalt the struggles of life [1]. When Yvonne Rainer showcased pedestrian, plain movement, she made a statement against the affective habits of performance, reflecting the sensibility of post Modern thought [2]. When Cynthia Oliver showcased black men onstage, she pointed to the dangers of race and gender stereotypes in inhibiting human expression and compassion, relating to a socio-political movement of her time: Black Lives Matter [3].
Thus, the utility of the theater is that it allows for a transcendence of the audience from their daily, conscious, real life to the spiritual, the unreal, the unconscious — or any other host of thematic venues: nature, struggle, and injustice. As a practical pursuit, the theater allows artists to hold up a prism to daily experience, transporting the motion of bodies into new, heightened contexts. The creation of this live, onstage fiction allows artists to show audiences new ways to think about familiar ideas — or familiar ways to think about new ideas.
Therefore, artists also have a task to complete in the process of incorporating robots into new, human-facing environments: telling new tales of new bodies in space to grapple with and roboticists have new measurements to take outside of the lab in varied contexts and themes for how people respond to robotic systems in varied settings and next to varied themes. The theater, then, is a setting where both parties can work together to present bridges between the fantastical and the normal and establish new conventions of moving machines in our environments. To get there, we must establish best practices for merging research inquiry with artistic exploration and collecting data from human audience members in parallel to theatrical experiences.
Perception of robots in the arts
A recent report from the International Federation of Robotics found that the number of worldwide in-home robots is growing quickly and may reach 31 million by 2019 [4]. However, acceptance, adoption, and consistent use of in-home robots requires an understanding of how robots are perceived by the general public. To this end, researchers have worked towards common metrics to measure aspects of human-robot interaction [5, 6]. The social and performance shaping factors outlined in these metrics have focused on measuring the affects robots have on the humans working with them or nearby.
Seminal work in HRI demonstrated that humans treat computers and other technologies as if they were human, while fully comprehending that they are not [7, 8]. Other studies have shown that humans apply these same social rules to robots [9, 10]. In addition to behavior, individuals’ perceptions may be influenced by the design or function of the robot, as well as the context in which it is presented [11–14].
Roboticists and artists have frequently collaborated on performance and research projects. The embedding of an experiment in a performance setting has been demonstrated by [15, 16]. Goldberg has asked questions about our relationship with technology through multiple artistic efforts [17, 18]. Burton and Kulic used Laban Movement Analysis (LMA) to create bio-inspired active sculptures that could be used in art installations [19]. Gemeinboeck and Saunders examined relationships between robotic art, performance studies, and computational creativity [20].
Egerstedt, Ludwig, and Murphy looked at difficulties in expressing narrative-inducing motion on nonlinear systems via a collaboration between roboticists and puppeteers [21]. Jochum has considered puppetry theater [22] as a medium for studying robots. Ikeuchi worked with dancers to control robots’ movements [23]. LaViers created a performance alongside professional dancers with the Softbank NAO robot to conduct exploration of style on moving bodies [24].
In work closest to the work presented in this paper, live performances with robots have employed the audience as research participants in order to study how elderly people perceive potential companion robots [25], to compare robot to robot and human actor to robot communications [26], and to experiment with robot control and character [27, 28]. As robots become more human-facing, this previous work combining artistic and robotic research endeavors provides insight on human perception of robots that would not be possible within the context of a research lab. On the other hand, technology for creating and capturing creative movement has also been explored. Cunningham used technology throughout his career [29, 30]. Schollig and Knight have worked to create tools for choreographers [31–33], and Fitters and Knight studied translations between actors and robots in [34].
One’s cultural background and prior experiences with robots influences their perceptions as well [35, 36]. A review of cognitive responses to robots shows that a robot’s form, movement, or present (virtual versus physical, for example) will influence the human interactant, in conjunction with the person’s own beliefs and experiences. Perceptions of robots as they appear in cinema and dramatic performance varied by demographics in [37]. Perceptions may also change before and after treatment according to the study measure, as shown in [38].
Perceptions of robots also varies by gender. Males were found to feel more positive about robots in healthcare than females did [39]. Women felt more negatively towards robots during human-robot verbal interactions [40] and when describing their sentiments about a future with robots [41].
Perception through the lens of expression
Roboticists have designed robots while considering the expressivity of the system [42, 43]. Tactics for programming existing robots with expressivity values in order to convey meaning appear in [44]. Methods for robots to display emotional and emotionally affecting body language were studied in [45, 46]. Thus, one measure of understanding individuals’ perceptions of robots is through the lens of expression. Merriam-Webster dictionary provides multiple definitions for the term “expression”, which has derivatives “expressivity” and “expressive”. These definitions are varied — from mechanisms in genetics to symbolic representation in math and in the arts. For this paper, the research team employs “expression” as a the capacity and ability to represent ideas through the medium of the body onstage, which is befitting of the context in which we explored the term, asking audience members about onstage bodies.
As part of the process of creating “expression”, humans can construct narrative from patterns in motion for stimuli where such attribution is surprising. For example, seminal work showed that simple shapes moving around on a plane can create consistent interpretation by human viewers [47]. The pattern of humans attributing socialness and animacy to robots is revealed in [48, 49].
However, audience members interpret any intended meaning of an artist through the filter of their own personal history, leading to individualized experiences for each audience member, at each performance. As individual experience is, by definition, subject to the individual, perceptions of self are embedded within perceptions of external forces and agents. Perception can be affected by many factors, including gender [50], choice of major [51], age group [52], quality of job [53], race [54], and body image [55], to name a few, all of which will be factors in HRI studies occurring inside theatrical contexts.
The interplay between context of presentation, an individual’s experience, and methods of abstraction appears in psychology (for example, why memories of the exact same event differs from individual to individual) [56], mathematics (for example, towards providing similar foundations when establishing new mathematical ontology) [57], and human-robot interaction (for example, in confronting challenges of implementing artificial cognition in social robots) [58].
Perception may also be influenced by the data entry modality itself. Different data entry methods (such as sliders, radio buttons, and the like) can create bias in outcomes as shown in [59].
Paper outline
With the goal of understanding existing perceptions of new technologies and how performative contexts can alter the perceptions of these technologies, we conducted a series of experiments studying audiences’ opinions of unfamiliar technologies, known technologies, various items, and human performing artists. Also included was the audiences’ perception of themselves. The experiments were conducted at four live performances in traditional proscenium venues. These four performances used the methodology outlined in [60] and extend the initial data presented in that paper. Details about the experiment structure, changes to the structure, characteristics of each instantiation, and symbiosis with artistic goals are presented here.
The research subjects were audience members who rated the expressivity of these items across different times of the performance. Providing the audience with the particular vocabulary of expressivity, through which to evaluate and experience a performance, is a central component of the study material. Factors that influence this idea include familiarity of each system, form of each system, demographics, and even how these systems have been presented in external environments, like popular media.
This section has outlined background on how the performing arts and roboticists have collaborated previously and on prior work investigating the perception of robot capacity. The paper will next describe the research motivations and artistic themes in Section 2. The paper will then outline early iterations of the performance/study structure that were abandoned or altered toward the end of collecting a useful dataset, including best practices, in Section
Then, the relevant results from the collected will be discussed in Section 4. Lastly, discussion and concluding remarks will be offered in Section 6.
Developing synergistic artistic themes and research questions
The work presented in this paper aimed to measure existing perceptions of robots, towards understanding how they may be perceived in the home, using design elements from performance in order to craft a rich, immersive experience that could mirror individuals’ earlier exposures to robots. The research team postulated that humans overestimate the capacity of artificial systems (especially unfamiliar artificial systems) and under-estimate the capacity of natural systems (such as humans). Moreover, exposure to robots may exaggerate this effect, creating wonderment at new, unfamiliar devices, which may carry a human shape.
Given the rich, numerous experiences and exposures to robots created by popular media, experiments that study perceptions of robots can utilize elements of storytelling, human-robot relationship, and designed elements, to recall similar feelings of viewing robots in these alternative media contexts. The process of crafting, editing, and presenting a performance is similar to the process of designing an experiment, towards the goal of recalling or altering a particular perception or behavior.
The team explored several lenses through which to try to measure this effect: power, valence, acceptability, and expressivity. The team then sought to evaluate how much, if at all, viewing a performance where technologies and
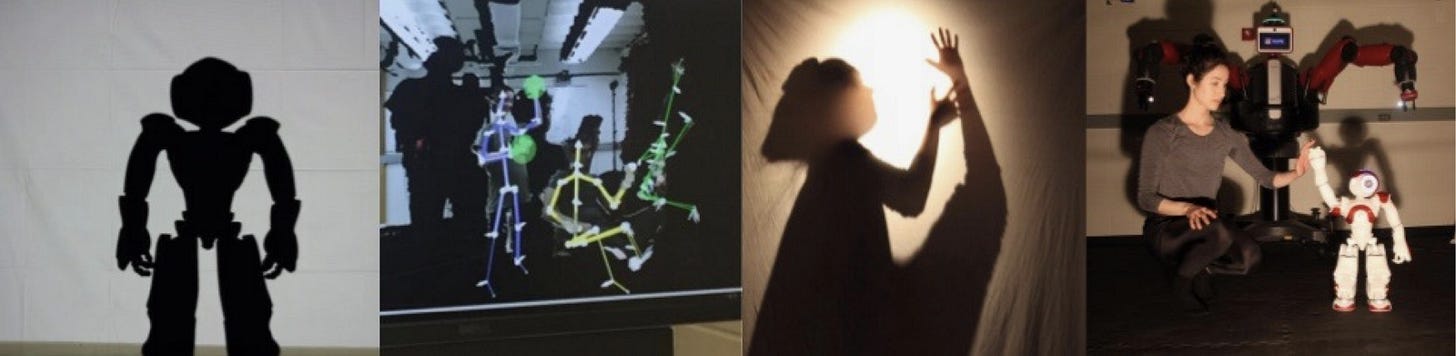
Figure 1
Figure 1: Images showing the themes of Time to Compile. On the left: an obfuscated NAO appears larger due to a projector behind it, projecting the robot onto a screen several feet away. Second from left: floating points of human bodies captured by the Microsoft Kinect. Second from right: a human altered by an overhead light and a sheet. On the right: a human performer moving with a NAO in the foreground as a Rethink Robotics Baxter stands in the background. These photographs portray the themes of Time to Compile in that they highlight the various ways human bodies are translated across and within pieces of sensor and actuator technology (the hidden human network). The dual shadows give a visual representation to the question, “Are humans becoming more robotic?” if humans are presented in similar contexts or environments as robots. On the far right, a human “compiles” their emotional, physical, and mental reaction to touching a robot
which could occur instantaneously and then mean something else much later, while it may take an indeterminate and often frustratingly long time for a computer to compile new information or a new program.
objects move alongside humans would change ratings of human and artificial system expressivity. In order to do this, an entertaining and though-provoking performance must be developed alongside a successful data collection procedure. Due to the challenges of collecting data in this live setting, discussed in the next section, we focused more on the latter goal, simplifying the set of lenses used to probe participants in favor of successful data collection.
The performance and experiment elements were created simultaneously over several months by a team of researchers and professional artists. A narrative inspired by this process began to emerge: one of a human user trapped in a cycle of mis-understandings and frustrations and one of a digital avatar suspended in the abstract universe of the Internet and machines. These two characters interacted through screens and devices (metaphorical and literal), before shedding their character types and recounting their own personal experiences with technology over the course of their lives. The design elements of the performance included choreography — for humans and the NAO robot (both the real machine and a projected shadow) — video, recorded music, dialogue, projection, and improvisation.
The developed piece, entitled Time to Compile and credited as a collaboration between an artist-in-residence and the research group, “Catie Cuan x RAD Lab”, examined the following themes, which are also illustrated in Figure 1:
Disjoint abilities in space and time facilitated by digital technology, e.g., holding live conversations on Skype across thousands of geographical miles and recording movements of a robot that will be replayed in a later task in a new context, respectively.
Constraints and capabilities inherent within natural and artificial systems.
The capturing of human data, e.g., the constant recording of human voice and face via the many personal devices that contain microphones and cameras.
Allusions to and desire for realness and familiarity — as exemplified by the NAO robot’s bilateral symmetry, roughly human proportions, and a high-sounding, “friendly” voice.
Establishing a spectrum of systems, reasoning about the relative capabilities of various natural and artificial systems, and the methods, such as spoken language or C++, necessary to create interactions between each.
These themes were a natural complement to the research aspects of the performance, as the experiment was focused at measuring perception through the simultaneous user studies, which will be described in the next section. Establishing a vocabulary for reasoning about these ideas — connecting the research instrument to the artistic themes — was necessary to probe audience perceptions about these ideas as well. The concepts “power”, “valence”, and “acceptance” were initially explored and eventually simplified to “expressivity”.
The title of the piece is a reference to differing compile times: the roughly instantaneous compile time of humans moving in improvised or newly created works, and the lengthy, error-ridden compile time of a program written for a machine. The credit of the piece is a recognition
of the dual contribution of each, as well as highlighting the symbiotic artistic and research (laboratory) efforts. In the end, a piece about programming robots became a way to ask audience members how they thought these machines worked with emphasis in contrasting live human actors to digitally controlled technology as well as ordinary objects. But, at the outset of this collaboration, different questions could have been asked, creating a different artistic expression and also a different lens through which to probe audience perceptions.
Study structure and data collection refinement
Across each of three performance venues, the Contextual Understanding of Robot to Audience Interaction (CURTAIN) framework, a performance-based experimental testbed for human-robot interaction first used in a performance at Brown University described in [60], was utilized to collect data for this paper. In the CURTAIN framework, an audience is primed for embodied interactions with robots by first viewing a performance and then interacting with robots in an embodied experience. Here, the experiments focused on performance priming only, without an interactive component. This interactive component of Time to Compile is described in [61–63].
The goal of this first component, live performance, was to understand how audiences perceive the various robotic, technological, and human agents in the show and then to isolate how the performance could alter these perceptions. The broad framework for this experimental setup is enumerated below and was refined over several instantiations.
The audience completes a pre-survey asking about their perceptions of various familiar and unfamiliar objects, in addition to humans.
The audience views a performance featuring the objects and humans.
The audience completes a post-survey, similar to the pre-survey, asking about their perceptions of the same set of systems through reverse-coded entry to prevent reliance on previous answers.
This paper reports on four instantiations of this framework, since the initial execution described in [60] where logistics of live performance were in tension with careful, controlled collection of data. In that prior work, we struggled to provide a theatrically interesting experience at the same time as successfully collecting a large data set. The challenge of collecting a large data set during a live performance was significant and the research team utilized the initial three performances to improve collection outcomes. Therefore a central contribution of this paper is the strategic refinement of survey collection platforms, narrative structuring, and personnel development to facilitate robust data collection for a human-robot interaction experiment inside of a live performance.
Aspects of the performance were modified to support the research experiment. The process of completing the surveys while watching a performance created a task for the audience that many experienced through the lens of success or failure (for example, wanting to rate the systems “correctly” on the expressivity scale). Audience members also expressed confusion or irritation about the survey task and felt that it detracted from the performance. Thus, compromises between artistic intentions and research goals created challenges as well as opportunities.
This tension existed at each performance and lead to several changes. Thus, the first three performances were primarily used to tweak collection and narrative elements to increase the number of audience members who volunteered for and completed the live surveys. The first three performances are included here to explicitly describe the changes to the experimental structure and the motivation behind those changes. Performance 4 is the only event that is described and discussed in detail for the remainder of this paper.
Performance 1: Ferst Center for the Arts, Atlanta, GA, April 21, 2018
The initial performance asked participants to select which entity was more powerful amongst various pictured pairings of the agents in the performance. The experiment shifted away from measures of power to measures of expressivity, as power is a more familiar topic and could be interpreted in vastly different ways. The survey link was printed in the evening program, leading to low rates of research participation. This was the largest venue and audience in the entire study with a venue holding over 1,000 seats and an estimated attendance of 400.
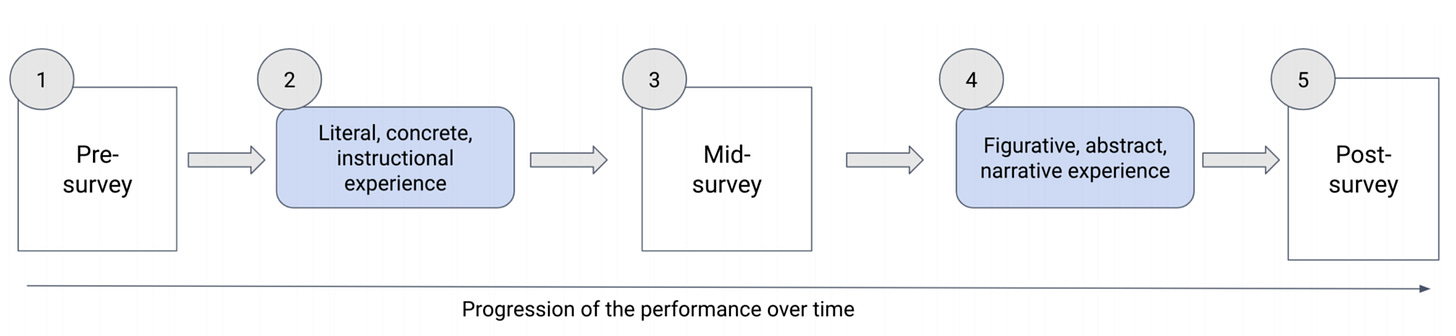
Figure 2: The experiment design illustrated from beginning to end. Three surveys were used through the performance to collect audience perceptions. Integrating the activity of filling out the surveys with the performance helped increase the number of responses and allowed for more points of feedback from audience members. Here, the first part of the performance is more concrete and literal than the abstract contemporary dance style used in the second part. This first section is used to homogenize the audiences’ understanding of vocabulary used in the study and point out subtleties about system construction.
Performance 2: The Midway, San Francisco, CA, June 6, 2018
An explanatory video for the notion of “expressivity” that the audience members should use was introduced, and a mid-performance survey was added. This survey acted as a second, or “informed”, pre-survey, where we hoped to homogenize the audience’s interpretation of the experimental instrument. The survey structure was altered such that participation in the survey was required in order for the narrative, and overall evening, to progress. This increased rates of survey participation and completion. The survey link was masked to incorporate the performance piece title, fostering a performative, rather than research associated, web portal. The number of survey questions was reduced. This was the smallest, but most crowded, venue in the study with roughly 150 attendees.
Performance 3: The Midway, San Francisco, CA, June 7, 2018
The ways of ranking expressivity from 1–10 were different across the three surveys to include check boxes, fill-in-the blank, and sliders. The tent cards were initially difficult to see from far distances, therefore audience members were encouraged to walk up to the stage to identify each item and label. This was the smallest, but most crowded, venue in the study with roughly 150 attendees.
Performance 4: Casa Paganini, Genoa, Italy, June 29, 2018
Ushers were introduced in the final iteration in order to assist audience members. This led to changes in the timings between the pre-survey and mid-survey, as the ushers checked the audience members’ progress. Overall, ushers increased rates of survey completion. This was a small professional theater, holding about 300 seats that was roughly
at one-third capacity, or 100 attendees who were also conference participants.
Final experiment design (Performance 4)
The final experiment structure, at Performance 4, is illustrated in Figure 2 and detailed below:
An audience arrives at the performance location and completes a pre-survey.
A short tutorial about the measure referenced in the survey instrument — in this case, expressivity — occurs. This element of the performance has literal, concrete, and instructional overtones.
The audience completes a second instrument, a mid-survey, with their new understanding of the metric in question.
The narrative component of the live performance is shown. This experience is a figurative, abstract, narrative experience, most reminiscent of a traditional proscenium standalone performance.
The audience completes a final post-survey.
At the start of the evening, audience members entered the performance location to find several items setup on the stage and labeled with tent cards (Figure 3). A slide projected behind the performers guided audience members to a web page where they accessed the pre-survey on their cell phones. An informed consent page appeared and audience members could elect to participate in the phone surveys or simply watch the performance without participating. These participants received the same holding pages and instructions, without receiving questions to fill in.
All surveys were accessed by waiting on intermediate pages in the survey until onstage visuals and audio instructed audience members (ushers also assisted on an individual basis) to advance the page. These intermediate
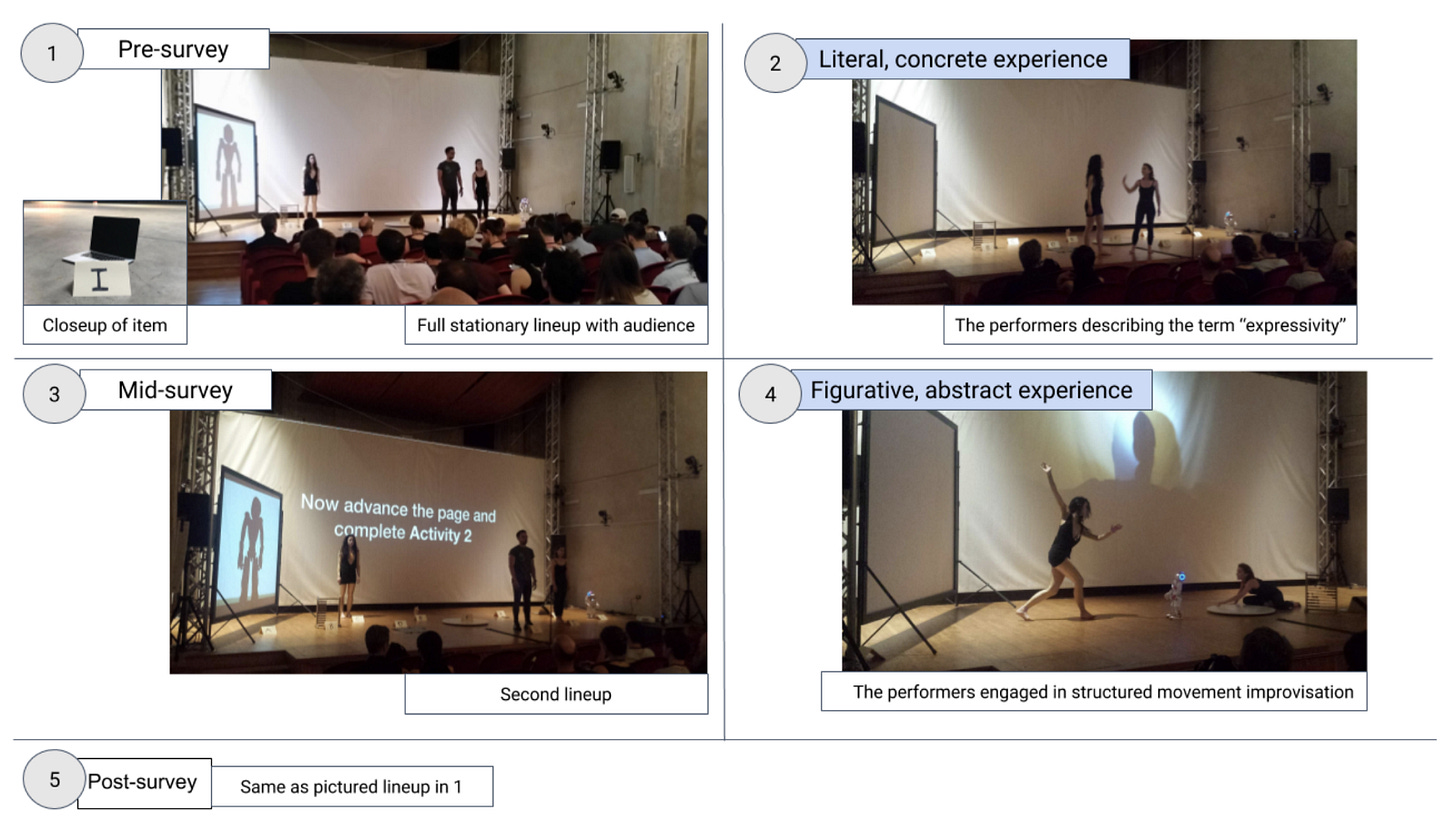
Figure 3
Figure 3: Pictures of the progression illustrated in Figure 2. In all images, Performer 1 is on the far left and Performer 2 is on the far right. The Male Audience Member is shown in the center of the two lineup images under Sections 1 and 3.
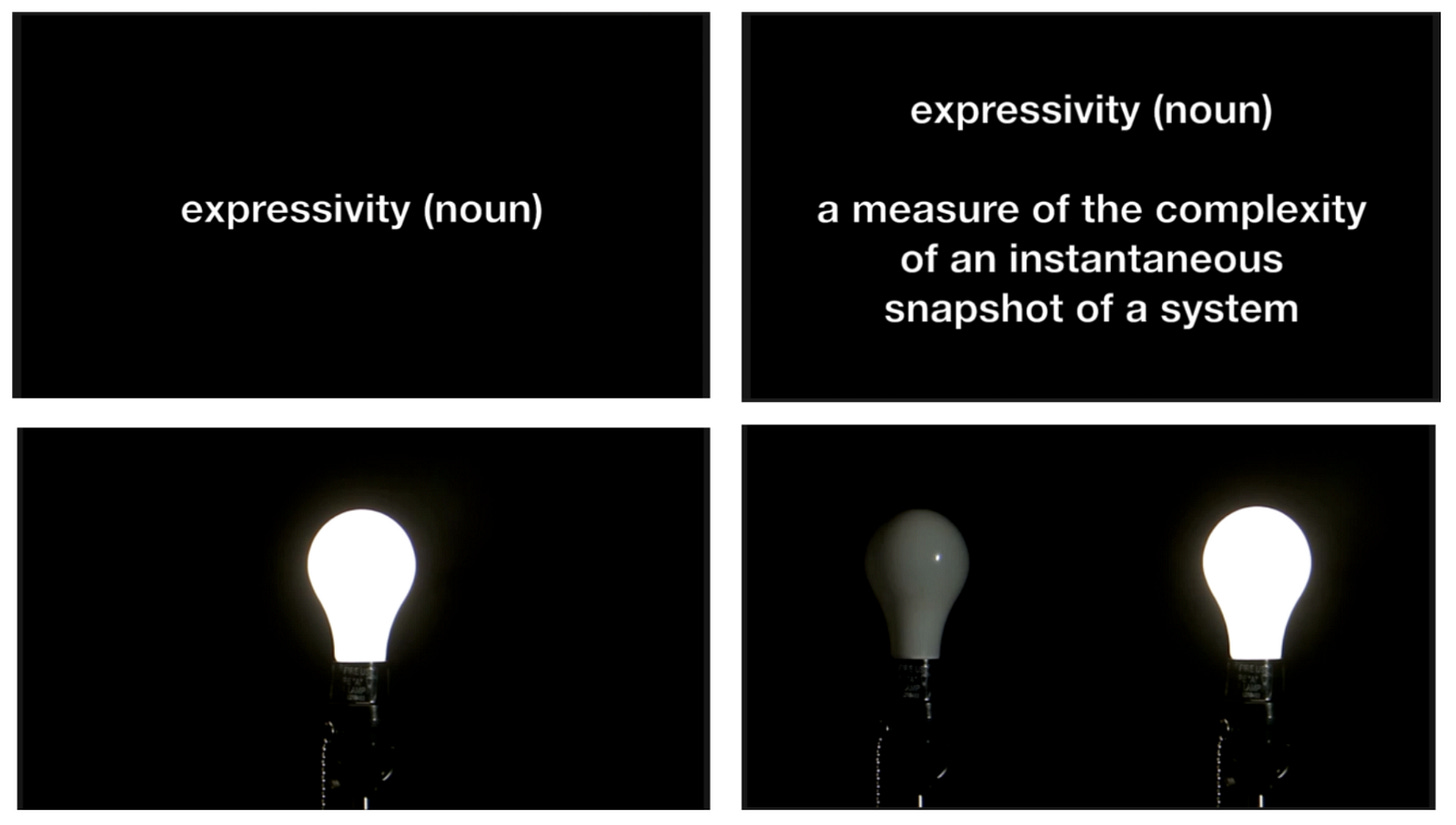
Figure 4
Figure 4: Images and the script for the concrete video experience in step two of the experiment. This instructional video solidified the definition of the expressivity measure between the first and second surveys with expressivity ratings: In the RAD Lab, we define expressivity, noun, as a measure of the complexity of an instantaneous snapshot of a system. Let’s look at an example. This is a light. It is a system. It turns on and off. It is an expressive system. These are two lights. They are a system. They can both turn on and off at the same time or out of sync. This a more expressive system. Let’s look at an another example… After this video, miked performers reenter for live explanation and demonstration shown in Quadrant 2 of Figure 3.
pages included theatrical elements as well as instructions to ensure audience members knew when to wait for further instruction from the stage before advancing the page. The first such holding page told the audience members to wait on the page until further instruction from the stage. The second such holding page was a video with a flashing light, which mimicked flashing light videos used on stage with both performers. This drew audience members into the piece through embodied action and repeated visual elements. Onstage performers, taking cues from ushers who were monitoring audience progress, did not advance to the next section of the piece until a quorum of phones where facing the stage.
The three surveys asked audience members to rate the expressivity of each system on the stage, from 1–10, with 10 being the most expressive, and then rate their own expressivity on the same scale. These participant ratings are the manner in which expressivity was measured. Performances 2, 3, and 4 included the same set of measured agents, namely: a NAO robot, a 2D large projection of the NAO robot, a laptop, a cell phone, an abacus, a t-shirt, a wooden hand, a spinning tabletop, Performer 1, Performer 2, a male volunteer, and the study participant themselves. Each item had a tent card with a letter labeling the system as “System A”, “System B”, and so on, as shown in the inset in Quadrant 1 of Figure 3. The audience members themselves were labeled “System Z”, to remain consistent with the system/letter naming convention used on stage. Each survey used a unique entry method: radio buttons, text entry, and a slider (with logic to ensure one integer between 1 and 10 was used).
In addition to the expressivity ratings, the pre-survey asked if the participant had an in-home robot, their likelihood of acquiring one in the next 5–10 years. The midsurvey repeated the expressivity ratings only. The postsurvey asked the previous two questions about owning an in-home robot, the expressivity ratings questions, and then prompted for a short written reaction to the evening’s events.
The onstage action proceeded as follows. The performers began in the line up shown in Quadrant 1 (upper left) of Figure 3, with projected instructions for accessing the survey behind them. Thus, audience members immediately began accessing the survey, with the assistance of ushers, as they walked in and found their seats. Once most had completed the initial survey, the objects were cleared from stage. Then, the explanatory video (Figure 4) played while the performers were offstage. The performers reentered and described expressivity through individual movements, partnered movements, and spoken text (Quadrant 2 of Figure 3). Then, the line up with tent cards was recreated (Quadrant 3 of Figure 3). Then, once most audience members had completed this survey, the objects were set for the top of the show with tent cards removed. The narrative component of the performance then began (Quadrant 4 of Figure 3).
After the formal performance was complete, the performers setup the “lineup” a final time and audience members advanced their web pages to the final survey. At the completion of the post-survey, the instructional page that instructed audience members to begin clapping was displayed. Therefore the ending of the performance was reliant upon several audience members reaching the final page and bravely breaking the silence by beginning to clap. Other audience members could be frequently found “sush”-ing this person or persons, erroneously thinking they were clapping in error. Eventually, enough would finish that the cue was understood by the group. When sufficient audience members were clapping, the performers bowed, signaling the end of the evening’s performance and experiment.
Thus, the resulting experiment was native to, and entirely informed by, the performance themes and structure. The research inquiry and performance work became intertwined such that the constraints, formalities, and procedures of each influenced the crafting of the other.
Results
The results of this data collection are two fold. The first challenge was in growing the size of the participant pool. Since encouraging audience participation required adjusting the format of the show, only the results from the largest participant pool will be presented here in detail. The following section also examines the structure of participant ratings on the variety of objects rated, revealing quantitative rating consistency with qualitative features of the onstage elements and performers.
Growing the participant pool size
There were a total of 54 study participants across four performances at three venues. Performances 2 and 3 were on consecutive evenings at the same performance venue. Performance 2 had an N = 15, Performance 3 had an N = 9, and Performance 4 had an N = 22 and an extended data set was collected this event. The overall growth in the participant pool size is shown in Figure 5.
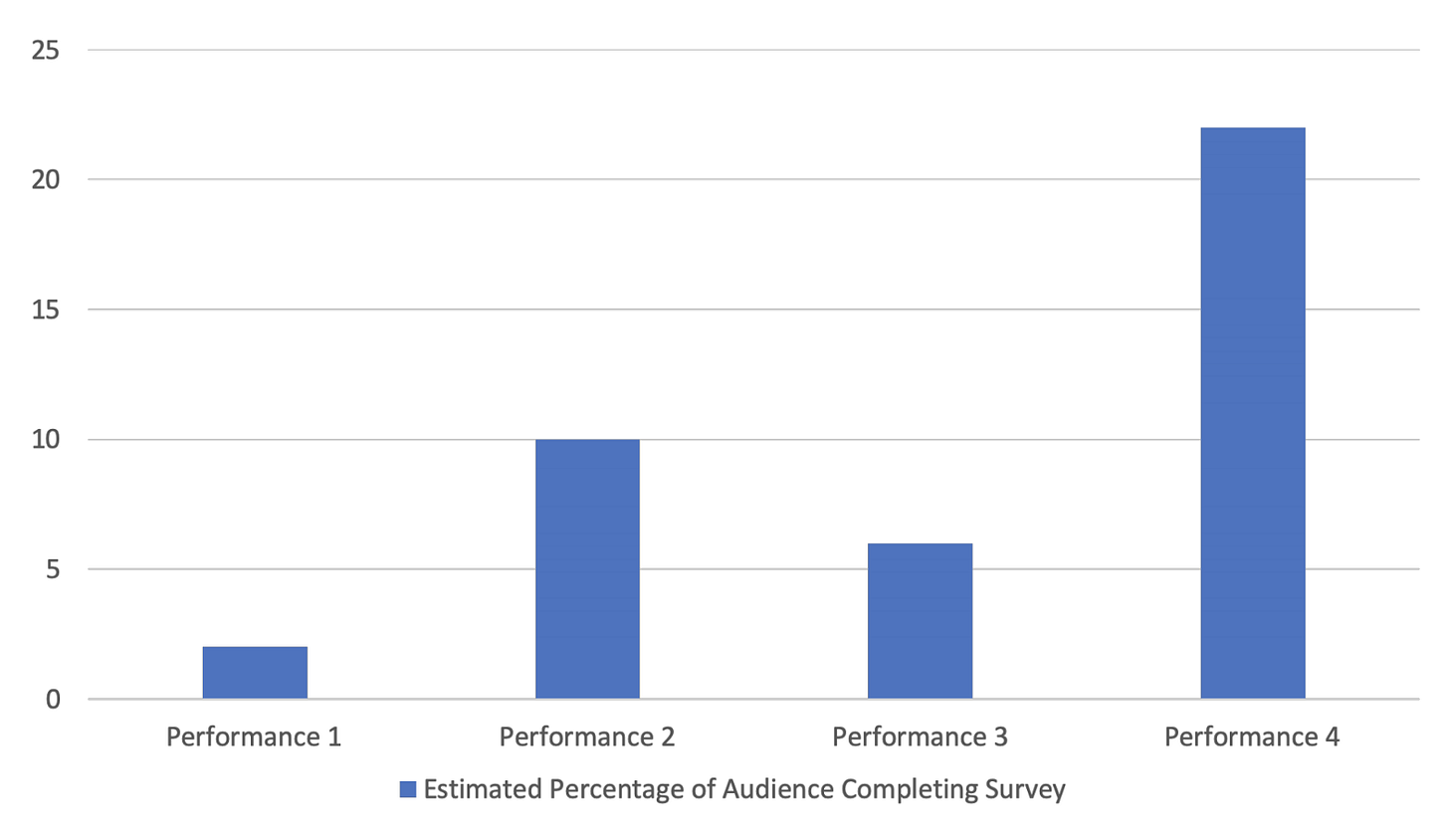
Figure 5
Figure 5: The estimated percentage of the audience at each performance that completed a survey, showing an overall increase, especially by Performance 4. Adjustments to the survey structure and number of audience members varied per evening, causing changes in the number of completed surveys across the performances.
Examination of the largest participant pool
This section, including Figures 6–9 and the tables in the Appendix, considers the largest participant pool, Performance 4. There was a total group size of 22 participants, 11 of which were male, 3 were female, and 8 were nonbinary or chose not to specify. 50% of the participants had a doctorate degree, the highest representation of any educational group (compared to Master’s, 4 Year Degree, Some College, High School Degree, Some High School). The average age of the participants was 35 years old. 7 participants were native English speakers, while Italian, French, and Other were the most likely other native languages, represented by 3, 4, and 4 individuals, respectively.
Tallying of the average Pre-Survey, Mid-Survey, and Post-Survey ratings for each system resulted in the distribution shown in Figure 6, calculated from a group size of 22 participants (the demographic make up of the pool is given in the Appendix). These systems have several attributes around which they could be organized, such as material makeup, number of moving parts, and artificial versus natural origin.
The instructional and theatrical experiences, including the film outlined in Figure 4, did not have a marked impact on expressivity ratings between the three repeated measures (Pre-Survey, Mid-Survey, and Post-Survey). The exception is the Male Audience Member which decreased significantly between the Pre-Survey and Mid-Survey. This implies that audience members may have an existing, consistent understanding of the idea of “expressivity”, that was not significantly affected by the instructional or theatrical experience, such that their original ratings persisted for the most part across each measure.
Although changes due to performative treatments were not significant, several trends are suggested in the data, which can be further explored in future work with larger participant pools. For example, average expressivity ratings increased for the NAO after audience members viewed the performance. Perhaps seeing the NAO’s movement range alongside humans who treated it as a lovable character, made it appear more expressive. However, the shadow of the NAO robot was rated slightly more expressive than the NAO itself, as the projector emanating the shadow image was clearly visible from the audience. This suggests that the size and scale of the projection may have led to increased perceptions of expressivity. The “Shadow NAO” average expressivity rating decreased after the performance, however, indicating that after prolonged exposure to the shadow, people realize the limited ability performers had to interact with this flat, pre-recorded image. Average expressivity ratings also increased for the Cell Phone after the performance, possibly showing that inclusion in performances, as the vehicle for audience participation, makes systems seem more expressive overall.
Moreover, the dataset of repeated “expressivity” ratings can provide a sorting of these natural and artificial systems. Three methods were used to understand the pattern in audience member ratings of the 12 systems: a principle component analysis, k-means clustering, and thresholding the average rating of each system. For the 12 items being rated, we considered 2, 3, and 4 groupings based on the structure of the dataset variance.
The spectral information of the co-variance matrix of the dataset of audience expressivity ratings was used to determine candidate groupings via principle component analysis [64]. Looking at the magnitude of the eigenvalues of this matrix, we determined that the first two components, comprising 41% and 20% of the data variance, respectively, contained the most information about the dataset. Using the eigenvectors associated with each of these components, we determined two possible groupings, shown in Figure 7, which lump humans and anthropomorphic, embodied technology into distinct groups.
A k-means clustering was also performed. Here, we used random initial clusters and performed the k-means algorithm with the built-in MATLAB function until clusters converged. The resulting groupings for 2, 3, and 4 clusters (the same number of groups as found through PCA) are shown in Figure 7. These groupings produce similar qualitative clusters: for two groups, humanoid and non-humanoid elements are sorted; for three groups, anthropomorphic agents are grouped separately from objects powered by computer chips and those which aren’t; for
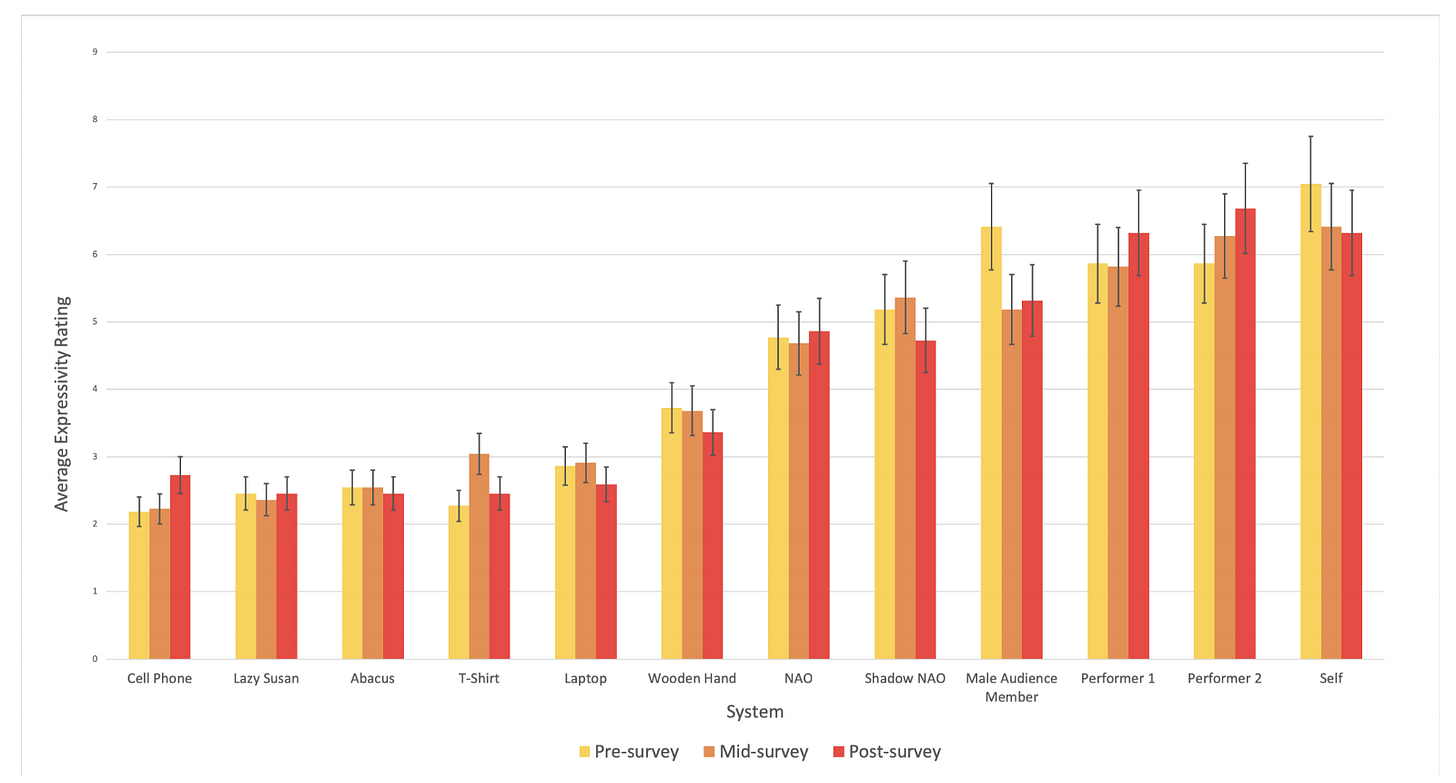
Figure 6
Figure 6: Average Expressivity Rating of all participants (Pre-Survey, Mid-Survey, and Post-Survey) for each System in Performance 4. Ordered by increasing overall average expressivity rating, humans (specifically Self) emerged as the highest rated followed by the humanoid elements used in the show (the NAO and NAO Shadow).
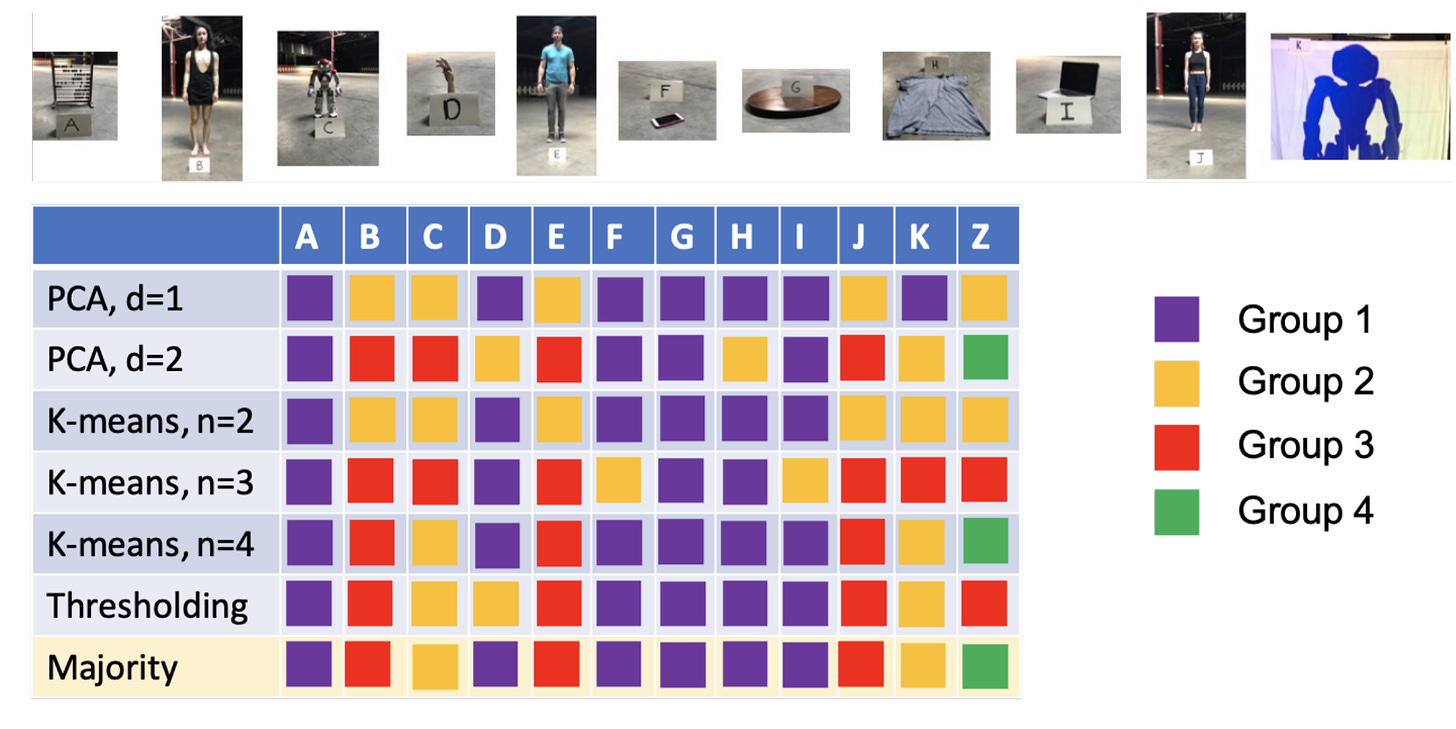
Figure 7
Figure 7: The line up of objects and the structure of ratings of each is presented in this figure. Top: Systems A-Z shown in the order they were presented onstage. Bottom: co-variance, clustering, and threshold analysis of the system ratings to determine groupings of objects that were similarly rated. We used three grouping techniques: principle component analysis, k-means clustering with random cluster initialization, and thresholding of averaged ratings (across Pre-Survey, Mid-Survey, and Post-Survey). For principle component analysis, the first dimension contained 41% of the variance and the second brought that to 61% with each component thereafter contributing less than 10% each; thus, organization according to these two components is presented here. An additional clustering method, k-means, was used for 2, 3, and 4 clusters with randomized initial seeding. Thresholds were determined that captured a similar structure. The results of all three methods show similar, but not definitive, structure in the data that is consistent with qualitative observations of system type according to the idea of “expressivity”. A majority grouping is suggested to capture the features of most of the prior clustering methods. While “Self” or “System Z” is the most inconclusive, having no majority grouping, it is always found in the highest order group, hence its assignment. This produces groups with the following consistent labels: objects, robots, humans, and self.
four groups, the “Self” system, which was most highly rated overall, achieves its own grouping. A simple thresholding, one of many methods used to explore the complex, ongoing research around clustering [65], was also performed to separate items with similar magnitude in rating. For this, we aim to capture the structure revealed by the other clustering methods, with a more straightforward interpretation (items with similar rating magnitudes go in the same group). Here we selected items with an average rating across the three surveys with: ratings < 3 as Group 1; ratings > 3 and < 5.5 as Group 2; and ratings > 5.5 as Group 3. Finally, since there are a myriad of clustering techniques with varying cluster number selection processes [66], a “majority” categorization was used to suggest a possible structure from this dataset. This categorization retains features of the other six candidate groupings, noting objects that were consistently clustered in the “higher order” categories. Under this method, Group 1 contained two objects always assigned to Group 1 (the Abacus and Lazy Susan), two objects assigned to Group 1 except in one instance or 83% of the time (the Cell Phone and Tee Shirt), and one object assigned 67% of the time (the Wooden Hand). Group 2 became two systems assigned to Group 2 in four of the six methods — or 67% of the time — (the NAO and NAO shadow). Group 3 became three systems always assigned to Group 3 when there were more than two groups and assigned in Group 2 in the two cases of only two clusters (the human performers and audience member). Finally, Group 4 became Self, which was always assigned to the highest order group. Thus, the categories resulting from audience rating at this performance are suggested as follows:
Objects: Cell Phone, Lazy Susan, Abacus, T-Shirt, Laptop, Wooden Hand
Robots: NAO robot, Shadow of NAO robot
Humans: Male Audience Member, Performer 1, Performer 2
Self
Changes in ratings organized by participant gender are shown in Figure 8. One trend is that expressivity ratings varied for different categories: the averages across gender groups cluster tightly for Objects, yet less so for Robots, and least so for People and Self. This could indicate that there might be greater disagreement between groups according to their gender for increasingly anthropomorphic objects, though the limited size of the participant pool eliminates tangible conclusions.
Women (out of gender choices female/male/choose not to specify) rated their own complexity the lowest (with a Pre-Survey mean expressivity of 5.5 and Post-Survey mean expressivity of 5.25). Men on average rated their expressivity 6.9 before the performance and 6.5 after. Nonbinary gender participants on average rated their expressivity 6.8 before the performance and 5.3 after the performance.
Qualitative feedback at different performances often referred to the idea of expressivity permeating the entire experience of the performance. Select quotations from Performance 4 are illustrated alongside their selected rating range and participant demographics in Figure 9. Additionally, qualitative comments from earlier performances gave insight into audience experience.
One audience member at Performance 3 noted, “Extremely interesting. Rating the different expressivities really forced me to think about how they operate, and I found myself going back and re-rating previous systems based on how I rated ones later on. Revisiting those rankings after the piece gave me a new perspective on their expressivity.” While participants could not go back in the survey, the term “revisiting” suggests that the experience of answering the survey questions over the course of the performance educated the audience about expressivity. Another audience member at Performance 3 wrote about how relationships could be viewed differently when given a vocabulary like expressivity, as another audience member wrote, “Humans are most expressive together”.
Discussion
Addressing the first challenge of growing the participant pool, several factors influenced the data collection while conducting research in this manner. The audience members needed to have a cell phone and Internet connection in order to participate, therefore survey participants excluded individuals who may not have brought a smartphone or known how to access the Internet. Survey participants may have discussed their ratings with the individuals sitting near them. Although participants were informed of the three coming surveys, they were unsure how long the performance would go on and therefore may not have had the patience to continue inputting responses.
Towards the second challenge of measuring audience member’s perception of the systems in the performance, across the Pre-Survey, Mid-Survey, and Post-Survey, there was the consistency in ratings of the systems into the following groups: Objects, Robots, and People, and Self. Given this sorting pattern, we conclude that the term “expressivity” is capturing perceptions of complexity but also
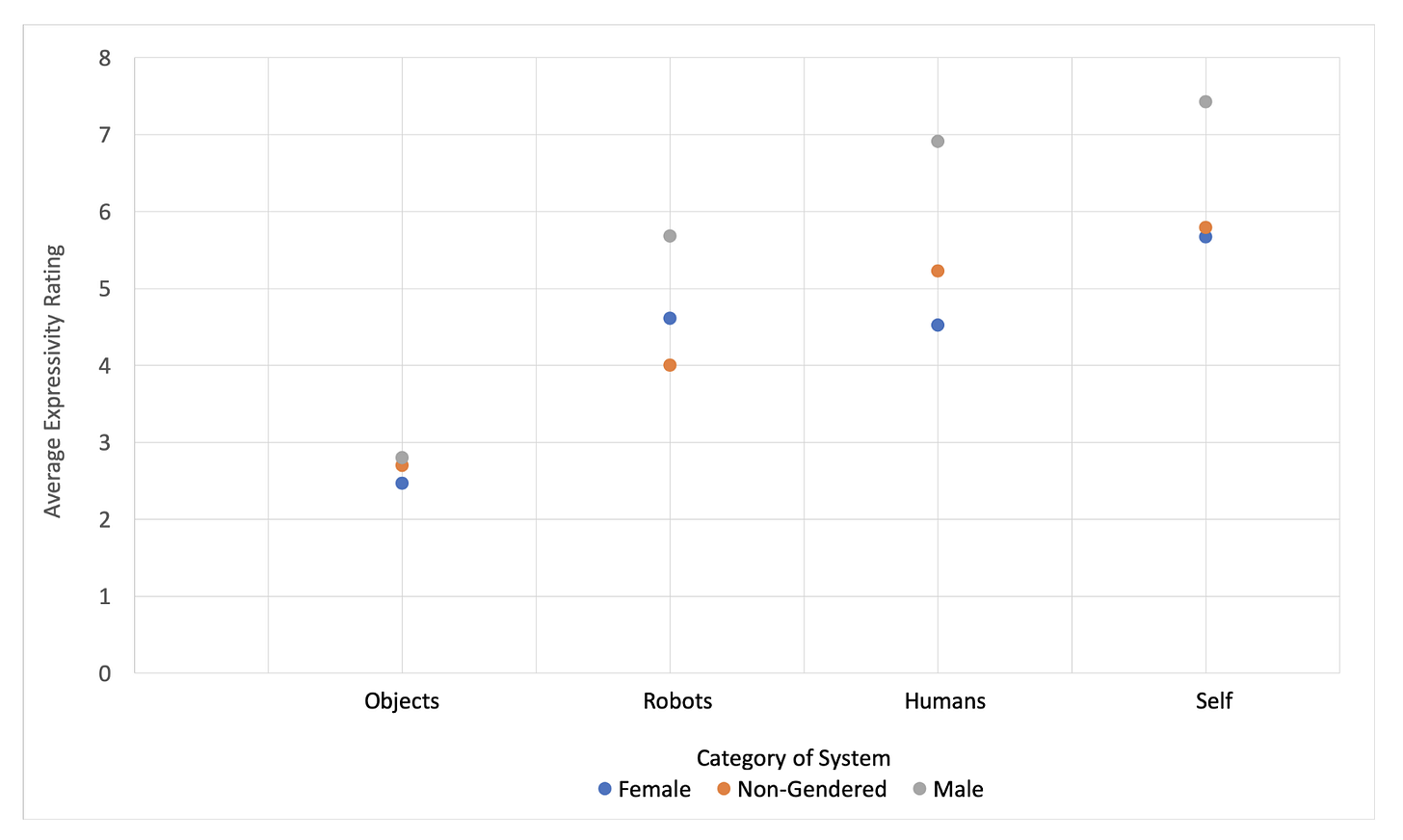
Figure 8
Figure 8: Average Expressivity Rating of 4 main System categories, sorted by a demographic feature, gender. All averages grouped closer together for Objects, while the widest range of average expressivity rating is for the category of Humans. For this participant pool size there was no statistically significant differences according to gender or any other demographic feature; however, this plot illustrates how the ratings for the human and robots were more varied — as is also reflected in the principal component analysis.
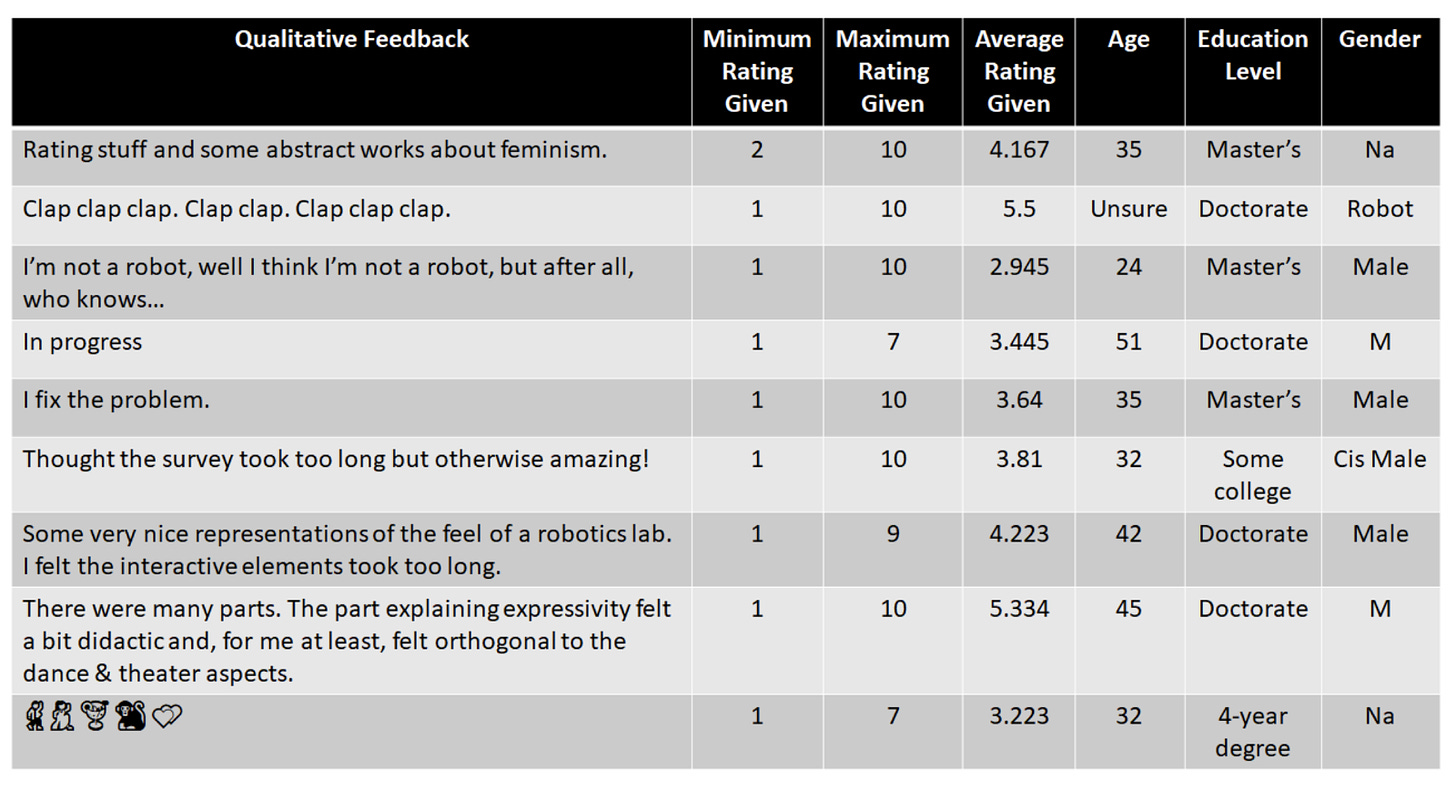
Figure 9
Figure 9: Qualitative Feedback from the User Study from Performance 4, in response to the question “Write a short reaction to tonight’s performance”, including general demographic and rating information of the participants who provided comments anthropomorphism. This demonstrates a perceived and understood difference between natural and artificial systems, though does not indicate other connotations with the term expressivity.
None of the performances were ticketed, meaning it is difficult to know the proportion of audience members that completed the survey. Performances 2, 3, and 4, while presented in a theatrical space, also had open entry and exit times, meaning the total audience numbers may have changed over time. While a ticketed event would have provided a clear count, it also would have been an expense on the audience’s part, which may have diminished participation rates further.
In the small Performance 4 participant pool, women were less likely to rate their own expressivity highly and men were more likely to rate robot expressivity highly. Across all participants, no person or object received an average score above 8.2 out of 10. The opinions of all performance items and agents infrequently changed before and after the performance, which poses additional questions about the malleability of opinions, study structure, and performance content.
Conclusions
This paper presented a series of experiments rooted in the previously developed CURTAIN model [60], utilizing live performance to measure human perceptions of various agents. Humans perceived other humans to be the most expressive, with anthropomorphic forms as secondarily expressive. Additionally, agents that appeared in the survey, like the Male Audience Member and Self, but did not appear in the performance, decreased in mean expressivity. This suggests that the demonstrated context of the live performance could be additive in contributing to perceptions of expression in objects in the show from the Pre-Survey to Post-Survey; however, further studies are needed to test this idea with a larger participant pool.
The organization of Objects, Robots, Humans, and Self show that individuals gather meaning from the term “expressivity” and perceive humans or human-derivative forms to possess greater expressivity. The scale of the various items (for example, the larger projection of the robot) may also influence the perception of expressivity. This raises further questions about individuals’ expectations of anthropomorphic artificial systems like the humanoid robot forms used in Time to Compile. Given the small number of participants, explicit conclusions about differences across genders cannot be drawn. However, differences in
self-perception and system-perception across any demographic groups would prompt further investigation with relevant psychology models, such as mental models of self, to glean how or why various technological systems may be adopted by different groups.
Future work includes measuring other perceptive lenses, like power, valence, and acceptability, in live performance. Additional design elements may be adjusted or employed in future performance iterations. The lesson section may be expanded to give further clarity to the survey participants. Increasing survey participation and completion is a continuous effort, towards reaching a show format where hundreds of audience members participate in a series of surveys over the course of the show. Further, perceptions of more natural and artificial systems, including additional robots and nascent technologies, could be tested. Other mediums, like online survey testing, may facilitate an increased number of participants rather than only those attending the live performance.
There are ongoing questions about the nature of the performance itself, and how much the abstract or narrative elements contribute to ratings changes versus sheer inclusion in a performance-type setting alone. Future experiments may isolate the measurement of the two core components the first being existing perceptions of artificial and natural systems and the second being the influence of a performance on these perceptions towards further identifying the salient trends of each. Such work, combining HRI experiments and theatrical production, may not only help understand perception of robots but also prove to be an important element in successful integration and correct function of these devices within the diverse breadth of human lives.
Acknowledgements: This work was conducted under IRB #17427 and supported by NSF grant #1528036 and DARPA award #D16AP00001. The authors thank Ishaan Pakrasi for co-creating and performing Time to Compile. The authors also wish to thank producers at The Ferst Center for the Arts, CODAME ART+TECH Festival, and MOCO 2018 for their support in presenting this work. Additional thanks to Cameron Scoggins for his vocal performance and to Alexandra Bacula, Roshni Kaushik, and Alexandra Nilles for ushering in Time to Compile.